Examining The Impact of Income - Expense Ratios on Bank Success Using Machine Learning Methods
Abstract
The study aims to examine the success of banks in the banking sector in terms of financial ratios. In this context, income-expense structure ratios for 24 selected banks operating in Türkiye for the period 2012-2022 were used. In the study, Net Operating Profit (Loss) / Total Assets was considered as the dependent variable. For each year of the 2012-2022 period, the average of the relevant variable was taken, and banks above the average were coded as successful, while those below were coded as unsuccessful. The examination of the period, especially considering the average taken for each year, allowed for the consideration of any emerging vulnerabilities or opportunities given the different cyclical conditions. Classification algorithms are used to determine which of the predefined classes a data point belongs to. The study predicted the 264 data points for the 24 banks for the period 2012-2022 using machine learning methods (Logistic Regression, k-Nearest Neighbors, Support Vector Machines, Artificial Neural Networks, CART, Random Forest, and Light Gradient Boosting Machine). According to the findings, the highest accuracy, precision, recall, and F1 score values among the machine learning methods used were shown by XGBoost, while the lowest values were shown by the KNN method. When examining the findings in terms of income-expense structure ratios, the most effective criteria for determining the success of a bank's operations were found to be total income / total expenses, net interest income after specific provisions / total assets, and other operating expenses / gross operating profit. The study found that banks maintaining a balance between income and expenses were successful. Among the machine learning methods used to determine the financial success of banks, XGBoost showed the highest performance. Additionally, the most effective criteria for assessing banks' operational success were identified, highlighting that these criteria are significant indicators for understanding bank performance. This study demonstrated the effectiveness of machine learning methods in evaluating the financial structures of banks and provided an important foundation for future research.
Keywords
Machine Learning, Banking Sector, Financial Performance
Full Text:
PDF
This work is licensed under a Creative Commons Attribution 4.0 International License.
Indexing and Abstracting Services
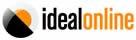
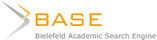
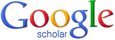
Other Sources and Services

License

Journal of Industrial Policy and Technology Management is licensed under a Creative Commons Attribution 4.0 International License.
Mailing Address
![]() | Journal of Industrial Policy and Technology Management |